Speeding up mechanical simulations with AI
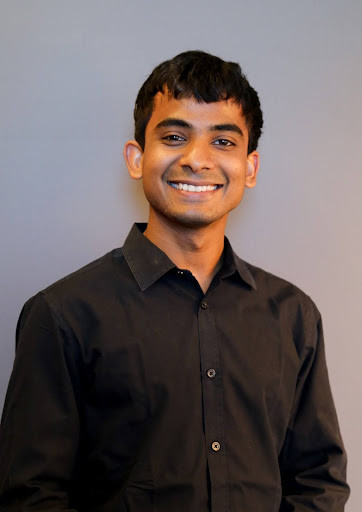
For five years, a single idea dominated Chinmay Shrivastava's thoughts: humans didn't have enough hours in the day to build the designs of the future. An avid lover of science fiction, Chinmay struggled to see how current mechanical engineering methods could build the starships that he dreamed would take him throughout the galaxy. While studying computational mechanics during his undergrad at IIT Roorkee, he watched a TED Talk in which Maurice Conti used AI to optimize a car chassis through millions of iterations. The resulting design outperformed what any human could have created alone.
But real-world engineering doesn't work that way, yet. High-fidelity simulations take days, cost thousands, and require hours of expert setup. Engineers are limited in how often they can test and improve their designs. Immediately after watching that TED talk 5 years ago, Shrivastava began working on AI models to accelerate simulation, but the early approaches, CNNs and LSTMs, couldn't properly model nonlinear physics or generalize beyond their training data.
He dreamed of a general model that could actually understand how physics affected 3D geometries.
In 2024, Shrivastava met Noah Evers while working on early LLM reasoning research. They began combining recent breakthroughs in physics-based learning with the paradigm of large-scale pretraining proven by ChatGPT. Their goal: to build a foundation model that could run complex mechanical simulations in seconds and extrapolate to new geometries, materials, and physical conditions.
Today, CompLabs is developing this model with leading aerospace, automotive, and manufacturing companies. These organizations regularly run simulations that take days and cost thousands of dollars.
CompLabs' model replicates the performance of an organization's existing simulation software, but in a version that runs in seconds, enabling engineers to explore broader design spaces faster and find better designs. These companies plan to use our model to test thousands of design permutations, like optimizing material and geometry combinations for thermal performance, compressing months of work into hours.
A technical and personal mission
Shrivastava's path to this problem was shaped by both expertise and personal pain. At 14, he suffered a severe reaction to carbon soot during Diwali. "I was coughing up blood," he recalls. "My hands and feet were covered in ulcers. I couldn't eat solid food for two weeks." He learned that carbon soot was a byproduct of design and manufacturing inefficiency and resolved to do something about it. This led him to study mechanical engineering.
"We waste energy everywhere," he explains. "Airplanes and cars burn more fuel than they need. Industrial systems—from HVAC to manufacturing equipment—are overdesigned and underoptimized. Everything is energy. And every bit of excess—fuel, material, or heat— translates into carbon. That carbon harms the planet."
After his carbon soot incident, he left home to become an engineer, studying in isolation for two years and attending IIT Roorkee and later Brown, where he worked on finite-element solvers, soft materials, and AI systems.
From speeding up simulation to intelligent design
CompLabs raised $2.65 million in pre-seed funding from Alt Capital, Cory Levy, and Joris Poort (Founder of Rescale). It's focused on building a new infrastructure layer for mechanical designs: one that uses foundation models to speed up simulations and reason about design trade-offs.
"We're not replacing solvers. Solvers serve the essential role of structurally approximating physics. We're just approximating these solvers in a more computationally efficient form."
But a model that understands geometries and how they're affected by physics has implications beyond simulation. It can autonomously improve designs and understand high-level concepts like manufacturability without relying on low-level heuristics. It can serve as a bridge between experimental results and numerical solvers, calibrating models to real-world data and even predicting system-level behavior (like force-response curves or failure modes) directly from shapes.
CompLabs hopes to use AI to supercharge the capabilities of mechanical engineers.
As Shrivastava puts it, "Mechanical engineering used to be glorious: steam engines, railways, airplanes, putting a man on the moon. Engineers were national heroes. But then software ate the world, and we lost that.
The times are changing. From SpaceX to Anduril, hardware is cool again. We're going to put datacenters in space, robots in the home, and fly on supersonic jets. AI is going to help us do that. We want to usher in a new golden age of mechanical engineering."
© Copyright IBTimes 2024. All rights reserved.