Preventing Artificial Intelligence Discrimination: Google Outlines A Strategy For 'Equal Opportunity By Design'
Artificial intelligence can be just as biased as human beings, which is why experts are trying to prevent discrimination in machine learning. In a new paper, three Google researchers note that there is no existing way to ensure—as the White House calls it—“equal opportunity by design,” but they have an idea.
“Despite the need, a vetted methodology in machine learning for preventing this kind of discrimination based on sensitive attributes has been lacking,” wrote Moritz Hardt, a research scientist with the Google Brain Team and co-author of the paper, in a blog post.
Hardt throws out two seemingly intuitive approaches, “fairness through unawareness” and “demographic parity,” but dismisses them for their respective loopholes. By learning from the shortcomings of the aforementioned methods, the team came up with a new approach. The core concept is to not use “sensitive attributes”—race, gender, disability, or religion—so that “individuals who qualify for a desirable outcome should have an equal chance of being correctly classified for this outcome.”
“We’ve proposed a methodology for measuring and preventing discrimination based on a set of sensitive attributes,” wrote Hardt, whose co-authors are his colleagues Eric Price and Nathan Srebro. “Our framework not only helps to scrutinize predictors to discover possible concerns. We also show how to adjust a given predictor so as to strike a better tradeoff between classification accuracy and non-discrimination if need be.”
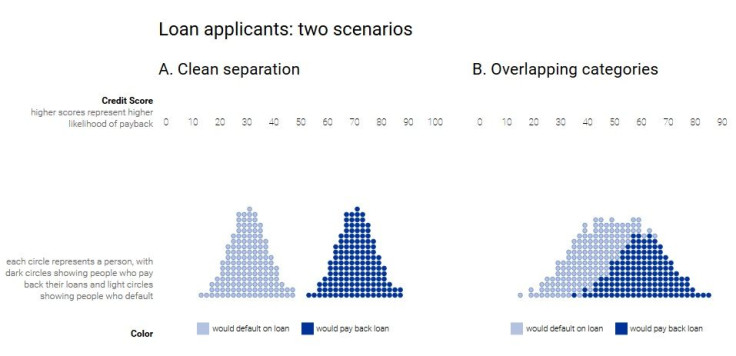
The researchers call this method of not including sensitive attributes equality of opportunity.
“When implemented, our framework also improves incentives by shifting the cost of poor predictions from the individual to the decision maker, who can respond by investing in improved prediction accuracy,” wrote Hardt. “Perfect predictors always satisfy our notion, showing that the central goal of building more accurate predictors is well aligned with the goal of avoiding discrimination.”
The equality of opportunity principle alone cannot solve discrimination in machine learning, as Google calls for a multidisciplinary approach.
“This work is just one step in a long chain of research,” says Google. “Optimizing for equal opportunity is just one of many tools that can be used to improve machine learning systems—and mathematics alone is unlikely to lead to the best solutions. Attacking discrimination in machine learning will ultimately require a careful, multidisciplinary approach.”
© Copyright IBTimes 2024. All rights reserved.