Cars On Google Street View Reveal US Demographic Makeup
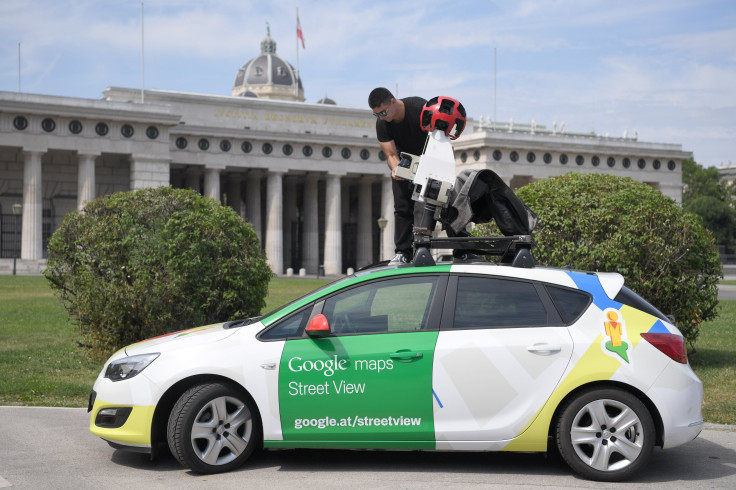
Conducting a survey of a population is not easy. It requires extensive manpower to produce the census data for the U.S. every year.
A Census of the population helps a government gauge the changes in population, new trends and how the population of a country form societies and co-exist.
It is estimated that the U.S. Census Bureau spends $1 billion surveying the population. The demographic makeup of any nation is revealed with the Census data alone, which is why it is done regularly.
The demographic study requires accurate and current data that will is very tough to produce on a regular basis. But now, a small group of researchers from Stanford University has come up with a very innovative, fast and higher-resolution way of studying the population.
Timnit Gebru and the team have tried to use Google Street View images to make remarkably accurate assessments of the demographic breakdown in a huge number of U.S. cities.
Their fascinating method involves studying over 50 million Street View images gathered by Google’s cars in 200 American cities over the years.
The team used an interesting parameter to gauge the demographic makeup of a location. They studied the type of car people owned in various locations as an indicator of their race, income, education levels, and occupation.
The team used an algorithm that can recognize cars in these images and then classify each in one of 2,657 different categories that depend not only on the make and model of the car but also its age.
This gave the team an accurate idea of how much the car is worth and a broad idea of the owner.
They studied nearly 22 million vehicles which amount to only 33 percent of the total cars in these 35 U.S. cities chosen for the study from the broader 200.
And it took their machine about two weeks to do the task at a rate of about 0.2 seconds per vehicle. “A human expert, assuming 10 seconds per image, would take more than 15 years to perform the same task,” said the team in a release by MIT Technology Review website.
To correlate the type of car and the demographic, the team summoned another deep-learning algorithm to understand the patterns using vehicle types and then check if it matched with data from U.S. Census and presidential election voting patterns in each precinct.
After the algorithm learned this information and combined the various datasets provided, the team set out to find out what it learned.
They found that the algorithm could use the pattern of vehicles in an area to predict demographics as recorded in the U.S. Census and presidential voting data.
“Using the classified motor vehicles in each neighborhood, we infer a wide range of demographic statistics, socioeconomic attributes, and political preferences of its residents,” the team were quoted as saying in the report.
“We found that by driving through a city for 15 minutes while counting sedans and pickup trucks, it is possible to reliably determine whether the city voted Democratic or Republican,” the team added.
The team also found that they could match car types with other demographic factors such as income, education, occupation, and so on.
According to the study published in Proceedings of the National Academy of Sciences of the United States of America, they were even able to make fine-grained predictions about voter preferences at the level of precincts, which encompass about 1,000 people.
© Copyright IBTimes 2024. All rights reserved.