Artificial Intelligence: MIT Researchers Create Deep-Learning Algorithm That Can Peer Into The Future
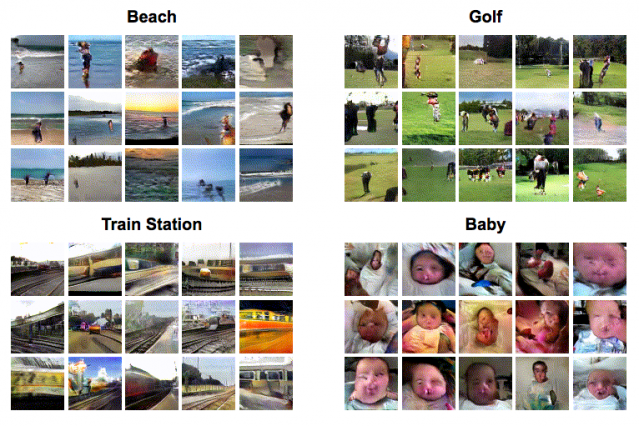
Despite the great strides artificial intelligence technology has made in recent years, machines are still woefully below the standard of general intelligence humans function at. One such area is prediction of future actions and events.
Imagine a man running on a racetrack. For humans with their cognitive faculties intact, it is extremely easy to foresee how the next few moments — or even minutes — would play out. For a machine, however, performing this action is a massive problem. If computers can be taught to understand how scenes change and objects interact, it would allow them to get a speculative glimpse of the future — something that is second nature for humans.
Researchers at Massachusetts Institute of Technology’s (MIT) Computer Science and Artificial Intelligence Laboratory have now managed to develop a deep-learning algorithm that can, given a still image from a scene, create a video that simulates the most likely future of that scene.
“These videos show us what computers think can happen in a scene,” Carl Vondrick, a PhD student at MIT and the lead author of a study describing the algorithm, said in a statement. “If you can predict the future, you must have understood something about the present.”
This predictive system was created using a deep-learning method called adversarial learning, wherein two neural networks — one that generates video and another that attempts to discriminate between real and generated videos — try to outsmart each other. Using this technique, the researchers were able to generate videos that were deemed to be realistic 20 percent more often than a baseline model of other computer-generated clips.
“In the future, this will let us scale up vision systems to recognize objects and scenes without any supervision, simply by training them on video,” Vondrick said.
One area of application that the researchers foresee is in self-driving cars. The technology can be used to create autonomous cars that are smarter and better equipped to deal with real-life situations, such as a pedestrian who is about to walk in front of the vehicle. Another possible use can be in filling gaps and resolving anomalies in security footages.
Of course, the technology still has severe limitations. For one, the videos it generates are extremely short — just one-and-a-half seconds long. This duration is something the researchers hope to increase in their future work.
“It’s difficult to aggregate accurate information across long time periods in videos,” Vondrick said. “If the video has both cooking and eating activities, you have to be able to link those two together to make sense of the scene.”
© Copyright IBTimes 2024. All rights reserved.