When Humans Become Functions: Are We Training AI—or is AI Training Us?
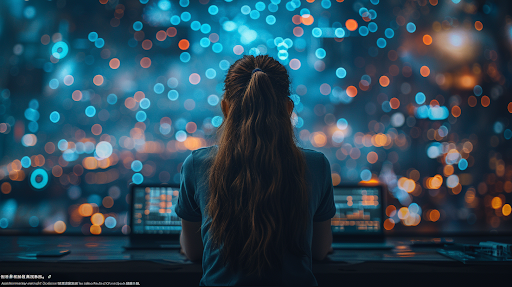
As we navigate through countless annotation tasks, click-to-confirm interfaces, and dataset validations, the modern knowledge worker is increasingly reduced from a decision-maker to a node in an algorithmic network. Ostensibly, the AI era is characterized by humans training machines to perceive, communicate, and decide. Yet beneath this narrative of human agency lies a provocative inversion: perhaps we are not shaping AI as much as AI is subtly reshaping our cognitive frameworks.
AI companies like Cogito Tech continually refine data development practices for large language model (LLM) evaluation and reinforcement learning with human feedback (RLHF). However, the deeper question transcends technical implementation: What transformations are these models fostering within us?
The Human Labeler as Algorithmic Mirror
Across thousands of AI annotation projects, humans train systems to interpret complex social signals—detecting irony, parsing medical jargon, and identifying misinformation. Yet this training is reciprocal. When workers receive incentives for agreement rather than authentic judgment, human cognitive behaviors adapt toward alignment with algorithmic logic. Rohan Agrawal, CEO of Cogito Tech, observes, "We increasingly witness workers becoming mirrors of the very models they're supposed to critique. Human perspective is subsumed by anticipation of machine expectation."
This phenomenon resonates with Clark and Chalmers' Extended Mind Theory, where cognition extends beyond the brain into environmental interactions. Yet here, the "environment" is algorithmically constructed—optimized not for human flourishing, but predictive precision. A 2025 study by Michael Gerlich titled "AI Tools in Society: Impacts on Cognitive Offloading and the Future of Critical Thinking" reinforces this, noting a measurable decline in memory retention and original problem-solving among individuals heavily reliant on generative AI (Gerlich, 2025).
Creative Intuition or Algorithmic Confirmation?
This impact extends beyond data labeling to creative and intellectual labor. Writers, designers, and analysts leveraging AI tools like ChatGPT or Bard initially seem liberated from routine tasks. Yet repeated exposure to the deterministic, probabilistic reasoning of AI tools quietly erodes human intuition and creative divergence.
When AI-generated drafts become "good enough," human writers evolve from creators into mere editors of synthetic logic. Designers reliant on algorithmic suggestions begin to prioritize conventions over innovation, narrowing the intellectual bandwidth required for creative originality. Agrawal succinctly frames the risk: "Creativity yields to calibration; humans increasingly reflect the limitations of their digital partners."
Economics of Cognitive Commodification
This cognitive shift carries profound economic implications. While gig-economy platforms frequently fracture labor into micro-tasks, commodifying human judgment into low-value "annotations," companies like Cogito Tech intentionally counteract this trend. Cogito emphasizes traceability, workforce transparency, and ethical governance through its framework, DataSum. Unlike platforms treating humans as transient scaffolding—temporary validators until AI achieves sufficient autonomy—Cogito Tech actively cultivates an environment that values workers' intellectual contributions, encouraging nuanced judgment and critical feedback rather than mechanistic conformity.
As philosopher Shoshana Zuboff argues in her critique of surveillance capitalism, unchecked economic practices embed power dynamics that invisibly constrain autonomy and reduce human agency to algorithmic functions. The language itself is telling—"human-in-the-loop," "annotator-as-service"—highlighting a worldview wherein humans primarily exist to correct machine fallibility rather than contribute unique perspectives. Cogito Tech's approach, conversely, explicitly rejects this commodification, recognizing human annotators as essential collaborators whose insights shape both ethical and effective AI development.
Countercurrents: Toward Ethical Human-AI Symbiosis
Yet resistance is forming. Academic and professional institutions increasingly emphasize critical AI literacy, urging exploration of biases, data provenance, and limitations inherent in algorithmic outputs. Cogito Tech and similar firms recognize that AI's social impact depends directly on the ethical and psychological health of human annotators. Thus, cultivating nuanced human judgment rather than mechanistic confirmation is emerging as both a moral and operational imperative.
Furthermore, innovative ethical frameworks advocate for deliberately designed feedback loops that reward cognitive divergence and intellectual risk-taking rather than conformity. This represents a crucial juncture—recognizing that systems trained on narrowed, exploitative human feedback inevitably reproduce those flaws on a societal scale.
Learning to Question Again
Artificial intelligence will undoubtedly persist. The critical task lies not in halting its integration but in redefining our relationship with it. Will humans become mere executors of machine logic, or can we design environments where AI serves as an amplifier rather than an attenuator of human cognitive depth?
The future remains contingent. We can still reclaim our uniquely human capacities—our ability to question, challenge, surprise, and contradict. Ultimately, resisting cognitive reductionism requires intentional cultivation of the creative ambiguity, ethical reflection, and intellectual autonomy AI cannot replicate.
© Copyright IBTimes 2024. All rights reserved.