Facebook And Artificial Intelligence: Company's AI Chief Explains How He Tags Your Photos
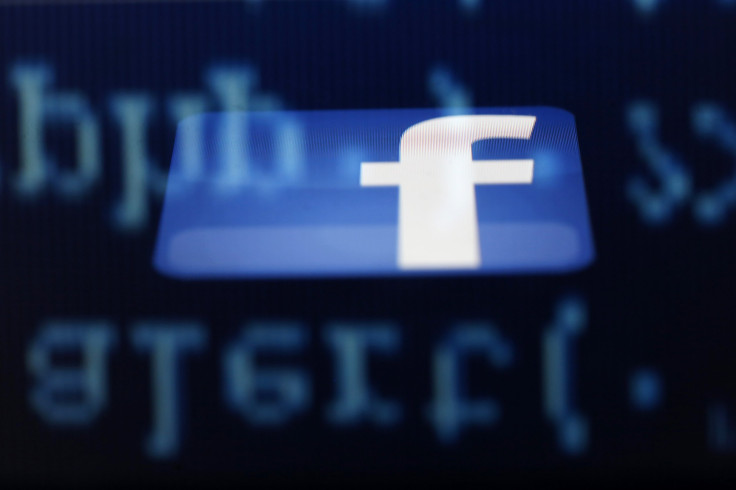
Google Inc. announced this month that it had developed the most accurate facial-recognition technology to date called FaceNet, which the company said trumped Facebook Inc.’s rival software called DeepFace by almost three percentage points in a test of accuracy. That was a tough truth for Facebook to swallow, because both companies have invested heavily in artificial-intelligence and computer-logic research to fuel the accuracy and speed of their respective systems, and because a billion monthly users already rely on a form of Facebook’s version to tag photographs when they log into the site. It appeared Facebook was getting beat at its own game.
Yann LeCun, head of Facebook’s Artificial Intelligence Research lab, spoke Tuesday about how Facebook originally built the tools that currently handle the site’s many photos and how his team plans to expand on that proficiency to build the next generation of artificial-intelligence software at an event co-sponsored by Facebook, Medidata and New York University’s Center for Data Science that was held at the former’s offices in New York. “It’s complicated, but it’s simpler than you might think,” LeCun said. He leads a 40-member group of artificial-intelligence experts that is only a year old, and split between Facebook’s offices in New York, the company’s headquarters in Menlo Park, California, and the firm’s new branch in Paris.
That team and Facebook’s developers are in a race against other major technological companies, including Google, to create the fastest and most sophisticated systems not only for facial recognition but also for a whole suite of products built on the tenets of artificial intelligence. Along with Facebook and Google, Alibaba Group Holding Ltd. and Amazon.com Inc. also have stated interests in this area, as Bloomberg Business reported. Last year, 16 artificial-intelligence startups were funded, while in 2010 the comparable figure was only two.
Facebook and its competitors believe people will increasingly rely on artificial intelligence to communicate with each other and to interact with the digital world. To stay ahead in this stiff competition, LeCun said his team needs to make breakthroughs in the field of deep learning, or the process by which machines can help humans at tasks that people have always proven best at, including making decisions or reasoning.
A computer capable of the advanced machine logic known as deep learning would require more inputs, outputs, levels and layers than Facebook’s facial recognition and photo-tagging software, but LeCun said both projects would rely on many of the same fundamental methods that computers and programmers currently use to organize and prioritize information.
At any given moment, Facebook software is busy tagging and categorizing the 500 million photos that users upload to the site each day, all within two seconds of when the images first appear. At nearly the same time, the system’s logic decides which photos to display to which users based not only on permissions but also on their preferences. Although the volume of data that this program processes would be mind-boggling for any human, the methods by which it sorts through those images are crafted by LeCun’s team.
Most Facebook users have seen friends’ names pop up in suggested tags when they upload photos to the site, but the company also uses tags to categorize the objects within images and help its software to decide which photos to display on the site. Although the system could display as many as 1,500 photos a day in a user’s stream, the average Facebook user will spend only enough time on the site to see between 100 and 150 images a day. A form of artificial intelligence helps Facebook ensure users are seeing the most important ones.
To create a similar system that would fuel the company's foray into deep learning, developers and experts began with a large database of images and tags such as ImageNet, and they built programs that learned to associate characteristics of each tag with specific types of images. For example, differentiating between colors and shapes helps the software pick out a black road versus a gray sidewalk in an image of a city street. “The network is able to take advantage of the fact that the world is compositional,” LeCun said.
Once the program recognizes features such as streets or sidewalks in a photo, it can draw a box around each object and identify them as separate from each other, or highlight examples of only one or the other. LeCun demonstrated this last concept in a shaky video taken on a walk through Washington Square Park in New York. The software picked out pedestrians as they moved past, drawing a rectangular box around them on the screen.
A sophisticated tagging program should also be able to first distinguish between a black road and a black car, and then assign names and categories to these objects. To do this, experts teach the system to grab contextual clues from the pixels surrounding an unidentified object to determine its most likely identity. So in that photo of a city street, the software may identify and tag a road based on its shape, its color and the presence of a nearby sidewalk. Then, it could surmise that the bulky shape in the center of that road is probably a black car.
When all these identification skills are added together, the resulting program can tag objects with some accuracy. In a demonstration, LeCun trained a video camera on a water bottle. A tag reading “water bottle” popped up at the top of the screen. Next, he panned over a beer bottle, a microphone and a laptop keyboard. The system correctly identified each. There were a few glitches, though -- the program identified his Samsung cell phone as an “iPod,” and when he panned over a plastic cup filled with red wine, it couldn’t decide between “red wine” and “beaker.”
Aside from shapes and colors, an ideal system must also rely on clues to help it assign specific tags to images related to activities, ideas or behaviors. For instance, LeCun showed a series of photos of people playing sports. A person who is rowing a boat is positioned very differently than a person who is jogging, and yet the system needs to recognize both athletes as people and categorize them by sport.
To break these different activities down to terms that a program might understand, data scientists focused on the angles of the arms, legs and torsos of the athletes who were engaging in various sports. They drew rods that spanned the length of the limbs of each athlete to help the computer track similarities in athletic positioning and identify the sport being played from image to image. Using this technique, the line for a baseball pitcher’s throwing arm would look very different than a line tracing a basketball player’s follow-through while taking a foul shot.
Computers can now distinguish between many activities by relying on such methods. One program that LeCun's team built can properly tag 800 sports, including “mountain unicycling,” “ice hockey” and “speed skating.”
Of course, the usefulness of Facebook’s photo-tagging software rests largely on its ability to recognize faces. The company trained its software to assess a face’s three-dimensional layout by running large databases of images through it, such as the 13,000 images contained in a set called Labeled Faces in the Wild, as IEEE Spectrum reported. Because not all photos are taken from the same angle, Facebook’s software repositions faces that are turned to the side and fills in key details such as eyes and lips to estimate what someone looks like from the front. The system isn’t perfect, but LeCun noted it can recognize far more people than the average person, even if the number of times it tags people incorrectly is slightly above average when compared with humans -- and even if the latest tests show that Facebook’s program lags Google’s FaceNet.
LeCun is optimistic his team will once again outpace Facebook’s competitors in the development of deep learning and artficial intelligence, but he is hesitant to state any explicit goals for his team’s progress. He seems to worry not only about risking his company’s reputation but also about the fact the entire field of artificial intelligence has weathered a series of long “AI winters” since the 1970s as its leaders have failed to deliver on hopes they once raised about flawless language processing and smart robotic assistants. With the daunting task of developing deep learning before him, LeCun is careful to enjoy the resurgence of interest in his field without raising hopes too high.
© Copyright IBTimes 2024. All rights reserved.