AI Hallucinations Show The Human Using LLMs Has An Important Role To Play, Say Experts
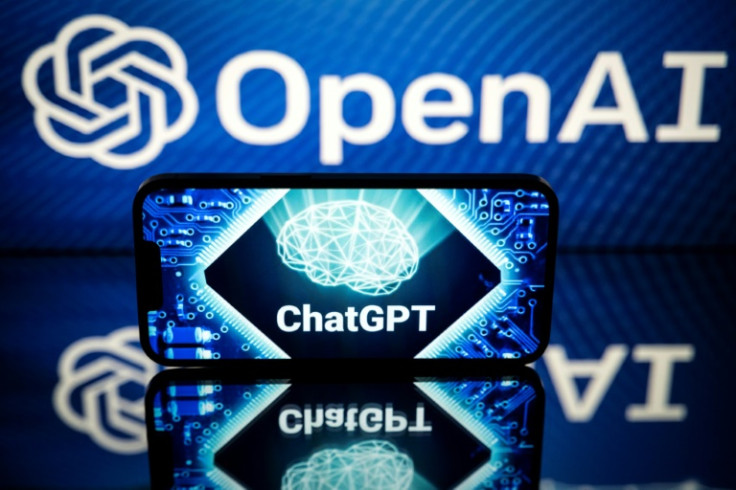
KEY POINTS
- 'Caveat emptor' – Latin for "let the buyer beware" – is applicable when using AI chatbots: ITIF's Daniel Castro
- Says Large Language Models might help people learn to question what they read and see
- People should "value themselves and make smarter decisions" when using AI systems: Reggie Townsend of SAS
- OpenAI has recommended a new approach for LLM training called "process supervision"
Even as generative artificial intelligence seems to be at the cusp of disrupting just about every industry, AI "hallucinations" have raised concerns, and raised questions about where the buck stops. Even more importantly, how to avoid the potential nasty consequences of the phenomenon.
But the answer to the problem may be very simple and could show why the humans that use the AI still have a very important role to play, interviews with experts show.
Reports have emerged of large language models, or LLMs, making "hallucinations," which is a term used to describe instances when AI chatbots generate false information. A recent example that grabbed attention was of a U.S. lawyer who asked OpenAI's ChatGPT to prepare a court filing, only for the chatbot to produce fabricated cases and rulings. The case raised questions about who was liable when a chatbot generated erroneous information, or -- in the human terms that AI has come to be portrayed in the media discourse -- simply lied.
Daniel Castro, vice-president of Washington, D.C.-based think tank the Information Technology and Innovation Foundation (ITIF) and director of ITIF's Center for Data and Innovation, told International Business Times that LLMs do not verify the accuracy of the responses they provide. The question who is at fault or who is liable may not just make sense, he said. "If someone uses the wrong tool for a job, we don't say the tool is faulty."
But first, there's the debate around the use of the term hallucination to describe the false outputs that AI-powered chatbots sometimes generate. Ars Technica's Benj Edwards wrote that "confabulation" – a human psychology term that implies the brain convincingly fills in a memory gap without the intent to deceive – may be a better metaphor, even if it is also an imperfect description.
But Castro said both terms "are misleadingly anthropomorphizing AI systems," considering how such systems do not think or understand and are only capable of generating realistic-looking outputs.
Reggie Townsend, vice-president of data ethics at analytics software and solutions firm SAS, said he prefers the term "inaccuracy," since most end users have different approaches in dealing with inaccurate information. Still, regardless of the descriptor used, human verification is crucial in AI outputs.
There is always a level of inaccuracy in AI model outputs, Townsend explained, adding that a chatbot's response is merely its "best attempt" to provide output based on its knowledge. Crucially, he pointed out that an LLM is designed to produce an output even when it does not have an adequate knowledge about the topics it is asked about. Better prompts or inputs can help reduce such hallucinations.
Castro, too, agreed that if prompts are adjusted, the AI's responses may be less likely to be false. And that verifying the information generated is necessary. He added that "caveat emptor" – a Latin phrase that translates to "let the buyer beware" – is applicable when using chatbots. At the end of the day, the end user is still responsible for ensuring that the AI's output is sensible and appropriate before it is used in a real-world situation.
Several experts have warned of the potential risks AI poses. Best-selling AI and tech author Bernard Marr wrote that false information from AI chatbots and systems present multiple issues such as erosion of trust, impact on decision-making, potential legal implications and ethics-related concerns.
In June, Geoffrey Hinton, one of the so-called "godfathers" of AI, urged governments to keep the technology in check. A month earlier, he said he decided to leave his position at Google so he can speak more freely about the dangers of AI.
ITIF's Castro reiterated that he believes no AI will ever have 100% accuracy, but he also said AI systems will get better and people will learn how to use the technology better through time. Instead of being a cause for major concern, AI hallucinations can serve as a good reminder that an authoritative response doesn't always mean that the response is correct. "LLMs might help people learn to question what they read and see."
He went on to note that hallucinations may be a "feature" of LLMs. He explained that LLMs may even see a "Wikepedia trend" -- it took people some time to discover that they shouldn't trust everything posted on Wikipedia but still, through time, the online encyclopedia has become an often reputable source even if it still has its drawbacks.
"It is important to not overreact to problems in new technologies. History shows that most things have a way of working out," he said.
SAS's Townsend said the challenge with chatbot inaccuracies lie within the end user. "We are prone to automation bias," which occurs when people trust automated systems over their own judgment even when the system, such as AI, is wrong. Having basic understanding of AI – both the opportunities and practical risks involved – will help reduce fear and increase understanding of why people should "value themselves and make smarter decisions" when using AI systems.
AI leaders have since recommended ways to help prevent hallucinations. OpenAI published a paper early in June to discuss a new approach to LLM training, which it called process supervision, as opposed to the old process called outcome supervision. The ChatGPT maker said that in the new process, an AI chatbot is directly trained to produce chains of thoughts endorsed by humans.
Data engineering company Innodata said placing additional constraints on a chatbot's responses, such as requiring the model to stay within known facts can also help reduce the likelihood of incorrect outputs.
© Copyright IBTimes 2024. All rights reserved.