Deepfake Videos: How To Detect Them?
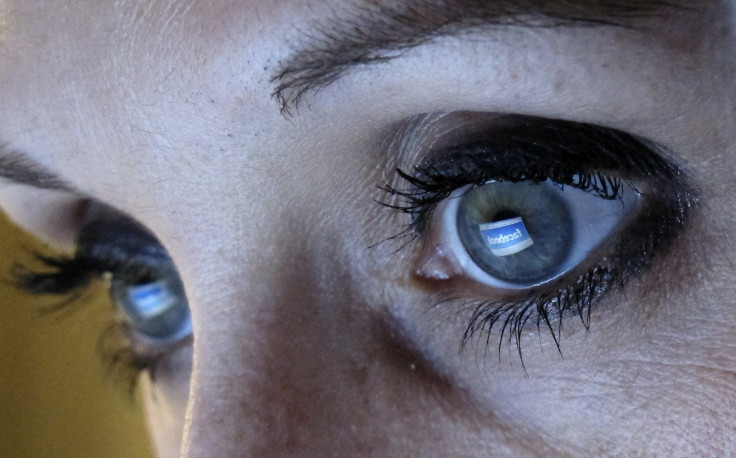
As WhatsApp, Facebook, and Twitter continue to adopt stringent policies against the spread of fake news, another form of misinformation is gaining traction from the masses — morphed videos known as "deepfakes."
These are extremely realistic face-swapped clips that can make a person — be it a celebrity or politician — say or do anything, without giving the real individual a clue of what is happening until it’s too late. The technology behind these relies on a deep neural network, a type of machine learning technique that learns from a source to produce a fake video of the target.
As part of the impersonation process, the neural network tracks the motion of a source face, including their way of speaking, and applies those characteristics to the facial image of the targeted individual. The resulting clip shows the targeted person doing or saying exactly what the source did.
The first deepfake came up in November 2017, and the ensuing months, a number of people have used the same technology to produce parodies as well as pornographic content using popular faces.
Prior to the rise of deepfakes, one could easily find morphed celebrity or politician videos on the internet. However, these particular clips are so realistic you won’t even notice a single sign indicating if a video is fake. This is why many think the same technology could be leveraged as a way to spread misinformation during the 2018 midterm election campaign season.
While there is no specific way to tackle the problem and identify a morphed deepfake video with 100 percent accuracy, Siwei Lyu, the director of Computer Vision and Machine Learning Lab at the University at Albany, and his team has found a temporary solution — a simple technique that might be used at least until the technology advances.
Detecting Deepfakes
The idea, as Lyu described, revolves around spotting how frequently the subject in the video blinks his or her eyes. Under normal conditions, a healthy adult blinks between 2 and 10 seconds and each blink could last somewhere around one-tenth to four-tenth of a second. This is exactly what one would see if a video is real.
However, if the clip is morphed, chances are that the rate of blinking won’t be normal or won’t be there at all. According to Lyu, who is a contributing author at The Conversation, the reason behind is the fact that most of the deepfake videos are created by training the neural network with loads of face images of the targeted individual in question.
In the case of celebrities and politicians, most of the images are sourced from the internet, where there are hardly any shots that show the person in question with eyes closed. Publishers mostly share images in which the subject has got both eyes open.
This affects the dataset fed into the neural network and is likely to result in a different blinking rate or no blinking at all. Lyu compared the normal blinking range with that seen in fake videos and found that the subjects blinked a lot less than real counterparts.
Lyu and colleagues even developed another machine learning algorithm to detect the blinking. The system analyzes a given video frame by frame to find the faces in it and if their eyes are open or closed. The method has already delivered promising result with a detection rate of more than 95 percent. However, with the advancement in technology, people making deepfakes could find a way to work around the problem and get better at making more morphed videos.
© Copyright IBTimes 2024. All rights reserved.