Coronavirus Update: Can Smartwatches Predict An Infection?
A person might experience disrupted sleep about 24 hours prior to becoming infected, according to a new study that analyzed data from medical wristbands. The study is, currently, undergoing its second round of review at the journal of IEEE Transactions on Biomedical Engineering.
The researchers used a machine-learning algorithm that used data collected from a medical wristband to analyze the sleep patterns of 25 individuals who had been exposed to a particular strain of the flu deliberately.
The findings revealed that, in a majority of participants who came down with the flu, the smartwatches recorded evidence of disrupted sleep 24 hours earlier.
While the research was initially focused on the flu, the researchers opined that this method can be general enough to spot the beginning of other infections including the deadly novel coronavirus.
"As we get more data from the populations wearing smartwatches through this pandemic, our predictive models will be refined. We imagine that these refined models could be used to generate an early warning signal and even potentially enable the prediction of asymptomatic spreading without tests," MedicalXpress quoted the study’s corresponding author Alfred Hero, the John H. Holland Distinguished University Professor of Electrical Engineering and Computer Science and the R. Jamison and Betty Williams Professor of Engineering.
Can this method be used to diagnose COVID-19? No, but it can provide some useful guidance about when a person should self-isolate or take other precautionary measures. Especially during this current pandemic situation, individuals experiencing symptoms of cold and flu are asked to self-isolate but there are still several patients out there who exhibit no symptoms at all. If such an algorithm can predict the likelihood of a person coming down with an illness, it can alert people to self-isolate themselves and can prevent asymptomatic patients from spreading the disease unknowingly.
“Our method can effectively detect sleep/wake states in the presence of sleep pattern shift. Utilizing integrated multisensor signal processing and adaptive training schemes, our algorithm is able to capture key sleep patterns in ambulatory monitoring, leading to better-automated sleep assessment and prediction,” concluded the study researchers.
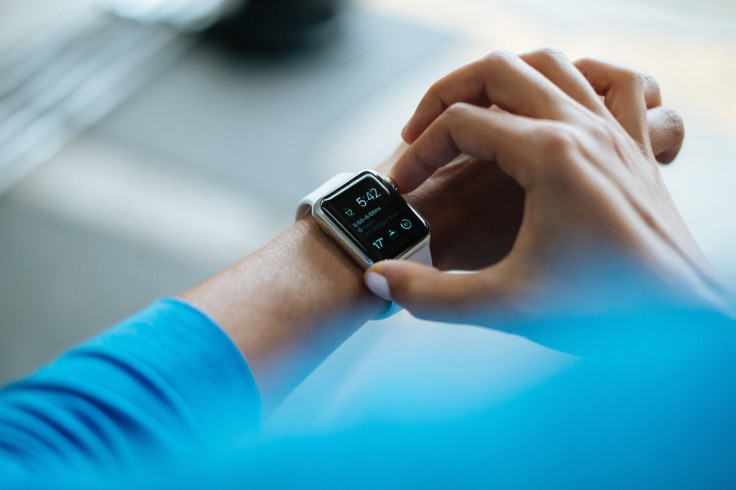
© Copyright IBTimes 2024. All rights reserved.